Accuracy Analysis
The accuracy of TRUST relative to other machine learning models is tested in 60 datasets: 20 synthetic (16 specially designed to challenge TRUST) and 40 real-world benchmark datasets (e.g. Abalone, Boston, Diamonds, Mpg, or Wine).
The alternative models are:
- M5', regularized linear model trees, similar to TRUST, by Wang & Witten (University of Waikato)
- Lasso, L1-regularized linear model, by Tibshirani (University of Toronto / Stanford University)
- NodeHarvest, a sparse rule extractor from a tree ensemble, by Meinshausen (University of Oxford / ETH Zurich)
- CART, the most popular piecewise-constant tree algorithm, by Breiman, Stone, Friedman and Olshen (UC Berkeley / Stanford University)
- Random Forest, the most popular tree ensemble model, by Breiman (UC Berkeley)
The first 4 are usually regarded as interpretable models, while the 5th one is a black box.
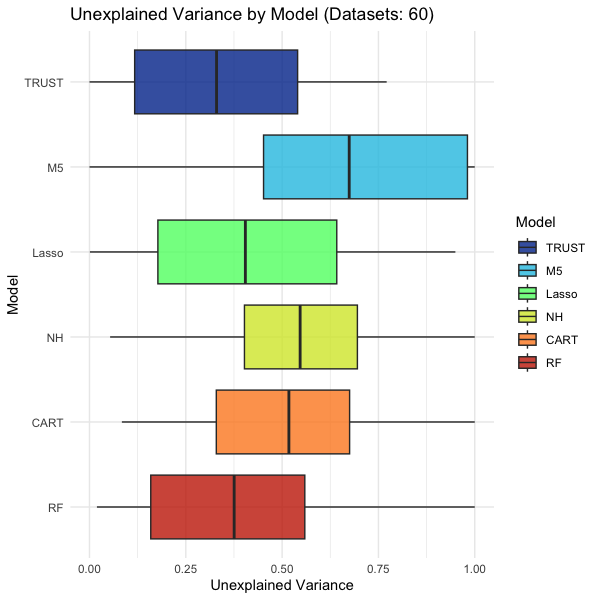
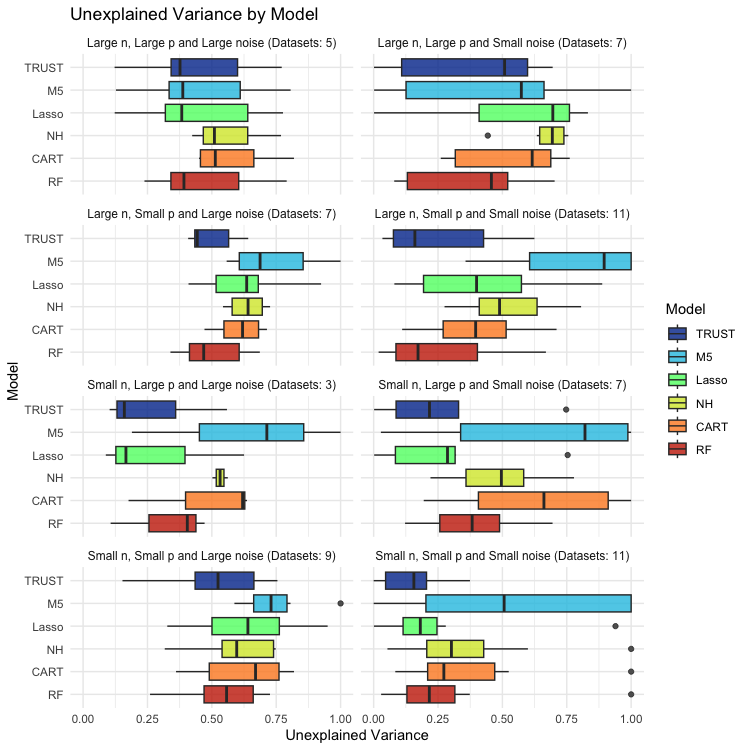
The first plot above shows that overall TRUST has comparable accuracy to a top-performing model like Random Forest (or slightly better), and tends to outperform other interpretable models.
The second plot shows a breakdown by dataset group, illustrating that TRUST is the only model that performs well under all conditions.
Lastly, other models have been included in a more comprehensive analysis (e.g. off-the-shelf XGBoost, deep neural networks or splines) and a similar conclusion holds.